Self-driving cars are set to revolutionize transport systems the world over. If the hype is to be believed, entirely autonomous vehicles are about to hit the open road.
The truth is more complex. The most advanced self-driving technologies work only in an extremely limited set of environments and weather conditions. And while most new cars will have some form of driver assistance in the coming years, autonomous cars that drive in all conditions without human oversight are still many years away.
One of the main problems is that it is hard to train vehicles to cope in all situations. And the most challenging situations are often the rarest. There is a huge variety of tricky circumstances that drivers rarely come across: a child running into the road, a vehicle driving on the wrong side of the street, an accident immediately ahead, and so on.
In each of these circumstances, a self-driving car must make good decisions, even though the likelihood of coming across them is small. And that raises an important question: how can carmakers train and test their vehicles when these events are so rare?
Today, we get an answer of sorts thanks to the work of Alexey Dosovitskiy at Intel Labs and a few pals at the Toyota Research Institute and the Computer Vision Center in Barcelona, Spain. They’ve created an open-source driving simulator that carmakers can use to test self-driving technologies under realistic driving conditions.
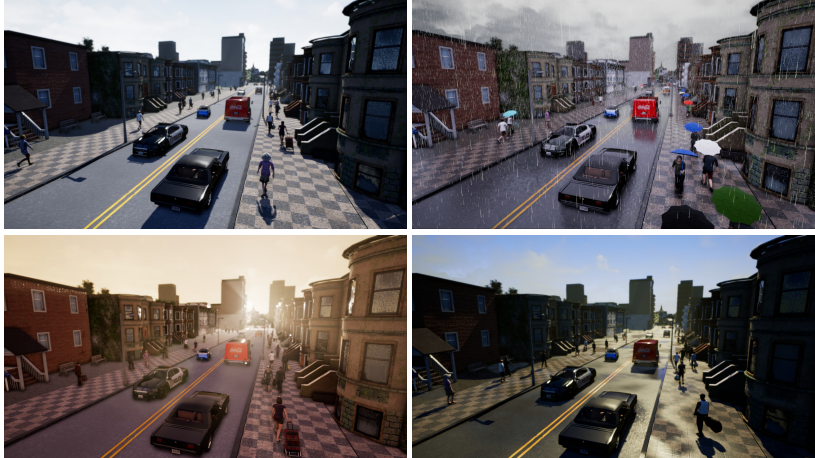
The system, called CARLA (Car Learning to Act), simulates a wide range of driving conditions and repeats dangerous situations endlessly to help learning. The team has already used it to evaluate the performance of several different approaches to autonomous driving.
Driving simulators are not new. There are numerous realistic driving and racing simulators, many designed for gaming. Various autonomous driving groups have used them to test their technologies.
But none of these simulators provide the kind of feedback that autonomous driving systems need to train effectively. Neither do these systems allow significant control over driving conditions or the actions of other agents.
Racing simulators do not usually have crossing traffic or pedestrians. And city simulators such as Grand Theft Auto do not give control over the weather, the position of the sun, the behavior of other cars, traffic signals and pedestrians, cyclists, and so on.
And these proprietary systems do not give the kind of technical feedback that autonomous driving systems need to learn.
So Dosovitskiy and co have created their own simulator. CARLA offers a library of assets that can be arranged into towns under various weather and lighting conditions. The library includes 40 different buildings, 16 animated vehicle models, and 50 animated pedestrians.
The team has used these to create two towns with several kilometers of drivable roads and then tested three different approaches to training self-driving systems. “The approaches are evaluated in controlled scenarios of increasing difficulty,” says the team.
The results show that the system can play a useful role. The team has published a video of the resulting driving behavior that clearly shows how well the systems can perform but also why this kind of training cannot be done on real roads—the cars sometimes drive on the sidewalk, on the opposite side of the road, hit other cars, and so on.
Of course, a system like CARLA can never replace driving time on real roads. But it can provide a useful and safe testing ground for new ideas. And that’s why it is important.
CARLA is open source and free to use for noncommercial purposes. So anybody can give it a go at www.carla.org. “We hope that CARLA will enable a broad community to actively engage in autonomous driving research,” says the team.
Source: CARLA: An Open Urban Driving Simulator